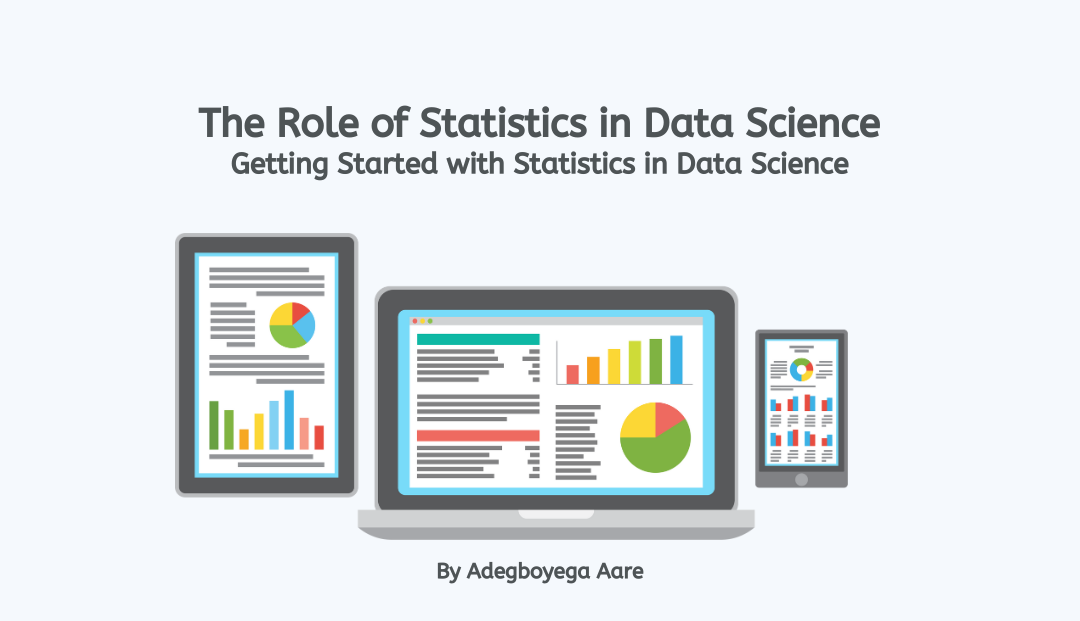
Statistics is a field of math that helps us work with data, whether it's numbers or descriptions. It's all about collecting, checking, and making sense of data so we can make smarter choices in business.
Statistics isn't just its own math topic; it's also a crucial part of data science. Using statistics in data science helps us uncover new insights, make better decisions, and even predict future trends.
In this article, we'll dive into how statistics is essential for data science and how it helps people who work with data.
Brief History of Statistics Usage in Data Science
For centuries, statistics has been a trusted partner of data science. In the 18th century, Carl Friedrich Gauss invented the least squares method, a big deal in modern regression analysis. In the 19th century, Francis Galton introduced correlation. But the real game-changers came in the mid-20th century when Jerzy Neyman and Egon Pearson created hypothesis testing, a super important tool in data analysis.
Then, when computers became a thing in the late 20th century, John Tukey and John Chambers came up with cool ways to visualize and explore data. Nowadays, statistics is still a big deal in data science. It helps us untangle tricky data and find juicy insights. It's like the trusty compass guiding us through the data jungle!
Current Role of Statistics in Data Science
Statistics maintains its essential role in the modern data science field. It assists us in understanding extensive data sets and extracting valuable insights. Here are some ways it does so:
1. Data Cleaning: Statistics aids in identifying and dealing with errors or outliers in datasets, ensuring data quality.
2. Descriptive Analysis: It allows us to summarize and understand data through measures like mean, median, and standard deviation.
3. Inferential Analysis: Statistics helps make predictions and draw conclusions about larger populations based on sample data.
4. A/B Testing and Experiments: A/B testing, a powerful technique in data science, comes into play.
5. Hypothesis Testing: It's vital for testing ideas and hypotheses, and determining if observed patterns are statistically significant.
6. Machine Learning: Statistical methods underpin many machine learning algorithms, guiding model development and evaluation.
7. Data Visualization: Statistics enhances data visualization, making it easier to convey insights to non-technical stakeholders.
Benefits of Statistics in Data Science
Statistics plays a crucial role in Data Science. It's like the secret sauce that makes all the data magic happen. Here are some benefits of statistics in Data Science:
1. Statistics helps Data Scientists make sense of the heaps of data they work with. It's like a flashlight in a dark cave, helping us see patterns and trends.
2. With statistics, we can make informed decisions. Imagine you're choosing between two ice cream flavours. Statistics can tell you which one people like more based on surveys, so you pick the tastier option!
3. Statistics helps us predict what might happen in the future. It's like looking at past weather data to guess if it will rain tomorrow.
4. Data Scientists use statistics to cut errors. It's like proofreading your essay before submitting it. Stats help us catch and correct mistakes in our data analysis.
Learn Statistics for Data Science
To start your data science journey, it's crucial to learn statistics. Here's a short list of YouTube videos to help you master statistics for data science:
For those of you new to my newsletter - follow me on LinkedIn, hit me up on Twitter and follow my Facebook Page.
To support me as I roll out more content weekly, click HERE to buy me a coffee.
This post is public so feel free to share it HERE, Thank you for reading…